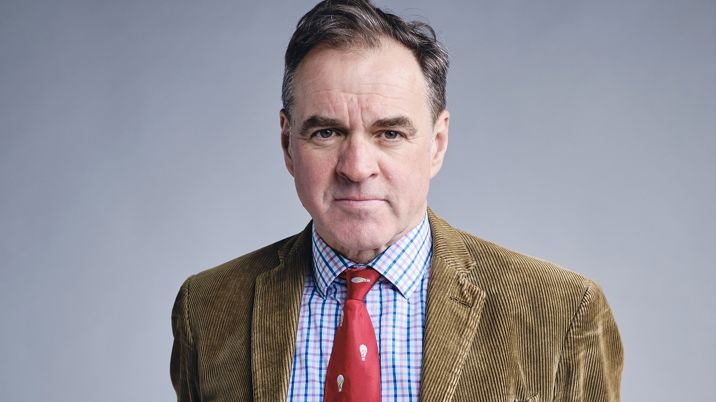
Sir Niall Ferguson Joins The Times
The Times announces Sir Niall Ferguson has joined as a contributing writer and will write 12 exclusive pieces per year. He offers his unique insights…
Thought Leader: Niall Ferguson
The emergence of artificial intelligence (AI) tools ushers in a groundbreaking opportunity in medicine. They have the potential to dramatically streamline drug development, broaden the spectrum of biological targets, and enhance the accuracy of diagnosis and treatment. For those who have engaged with the expansive capabilities of the large language model ChatGPT, it is not hard to conceive of the seismic shift these AI tools will trigger in the practice of medicine.
However, integrating these technologies into the current regulatory frameworks presents a considerable challenge. Global regulatory bodies, including the US Food and Drug Administration (FDA), will grapple with the task of applying their established norms to these novel entities. Consequently, new policies are needed to ensure the safety and efficacy of these tools for patients. These fitted solutions must balance the need for innovation with that of patient safety and benefit.
Artificial intelligence has the potential to help solve some of the most frustrating problems in health care. Clinicians may use it to stratify patients more precisely according to their personal risk and to identify increasingly tailored treatments that simultaneously account for a patient’s clinical history, genomic profile, and phenotypic characteristics. The combination of statistics and weighted observations in a neural network can be highly predictive. This is true even though each output for an individual patient is likely to differ from any other patient in a validation model, and even though the variables, when taken individually, are not likely to be nearly as predictive.
Artificial intelligence can also help transform binary, objective end points into statistical metrics of disease severity that are much more predictive; for example, providing a more quantitative measure of heart failure rather than relying on a composite measure from a small number of objective outputs such as left ventricular ejection fraction and exercise tolerance. Artificial intelligence can translate a larger universe of genomic, proteomic, and phenotypic data into a more complex assessment of risk, improving clinical trial design to select patients who are more likely to benefit from a treatment and—using the same tool in clinical practice—refining stratification variables for effective prescribing decisions. Another example is cancer treatment. Artificial intelligence can help develop models that examine minimal residual disease using large quantities of phenotypic data to better predict the likelihood of cancer relapse, allowing for more targeted drug development and effective treatment decisions.
The FDA has recognized the significant role that AI can play in drug development and software-based medical devices and is working to establish an appropriate framework within its existing authorities. Traditionally, the FDA evaluates and authorizes medical products in prospective trials based on very specific criteria and clinical trial data demonstrating a therapy met objective and transparent end points. Artificial intelligence presents a unique challenge to this established model. Relying on predictive measures of risk that exist on a graduated continuum, AI tools assess risk and benefit based on their own neural network through a process that regulators cannot decouple. Therefore, the best way to assess the safety and reliability of AI, in any reasonably sized clinical trial, may be to test it against historical events to confirm whether it is able to make predictions based on inputs when the outcomes are already known.
To foster innovation and ensure the safe, effective incorporation of AI in drug development and medical practice, the FDA will need to adopt regulatory policies tailored to the unique challenges and opportunities that these modern tools present. In certain instances, this effort may require Congress to grant the FDA specific authorities to establish properly tailored policies. Contrary to the FDA’s conventional regulatory approach, which is typically retrospective—evaluating the safety and efficacy of products after they are fully developed—AI regulation may require an approach that more closely resembles the new authorities that Congress granted the FDA to regulate over-the-counter drugs. This process involves defining prospective criteria that guide the safe development of products, and then ensuring that new entrants adhere to these established standards.
For example, the FDA could establish a framework for determining the appropriateness of the data sets on which AI tools would be trained. The agency could develop specific criteria for creating suitable data sets, assessing them for the breadth and reliability of the inputted data, the completeness of longitudinal phenotypic data, and the representativeness of the data set. It is essential to ensure that such data sets have been carefully curated to be broad and inclusive, representing the natural diversity of the general population.
The FDA also needs clear criteria to ensure that the benefits of AI tools can be accurately assessed. The potential benefits may be unlike those that the agency has considered for more traditional digital diagnostics and early generation AI tools such as locked AI devices that primarily read medical images. A significant benefit of the more complex AI models being developed from a wide array of health care data is their ability to analyze a wide range of heterogeneous sources, thus producing more targeted insights and recommendations. The agency will need to account for the benefits of tools that incorporate new combinations of data that could not be evaluated at scale before the advent of more advanced AI. Another advantage of these tools to consider is their capacity to evaluate a much larger population of people more quickly than with traditional diagnostic tools. The FDA should consider weighing the benefits of certain AI applications by viewing them as population health tools, which may enable the clinical community to understand more precisely the clinical needs of hundreds or thousands of patients within a short period.
Properly managing the potential risks will also necessitate new regulatory approaches, recognizing that the output from AI tools may be informative rather than determinative. For applications incorporated into drug approvals or serving as standalone medical devices, regulators could accept narrow indications with labeling that helps users understand the limitations. A suitably tailored framework should also consider the intended influence of AI output on clinical decision-making, incorporating flexibility. This framework would include giving due consideration to circumstances where clinicians apply their own expertise and judgment in conjunction with an AI-generated output.1
The fusion of large language models with the realms of science and medicine is already in motion. This integration can help catalyze a rapid advance in the efficiency of drug development and the precision of medical care. To advance these opportunities and properly assess the application of AI to medicine, a regulatory framework must be properly suited to the unique attributes of these technologies. That may require the FDA, perhaps with help from Congress, to craft a modern framework from the ground up rather than try to retrofit one of its existing paradigms onto these novel innovations.
Sir Niall Ferguson Joins The Times
The Times announces Sir Niall Ferguson has joined as a contributing writer and will write 12 exclusive pieces per year. He offers his unique insights…
Thought Leader: Niall Ferguson
Dr. Sanjay Gupta: Is Ozempic Really A Miracle Drug?
New drugs like Wegovy, Ozempic and Mounjaro are billed as a revolutionary new approach for losing weight. But are these actually miracle drugs? Dr. Sanjay…
Thought Leader: Sanjay Gupta
Peter Zeihan: The Geopolitics of Climate Change
From Peter Zeihan: With a glacier as the backdrop for this video, I figured it only appropriate to discuss resource exploitation, Arctic shipping, and agriculture…
Thought Leader: Peter Zeihan